Researchers in Prof Jeffrey Barrick’s laboratory (University of Texas at Austin, Austin, TX, USA) use experiments with microorganisms to study evolution in the laboratory as it happens. The team investigates a variety of biological mechanisms that can influence evolutionary processes ranging from changes in mutation rates to expanding genetic codes with unnatural amino acids.
One line of their research develops methods to track rare mutations in an evolving population to assess effects on fitness from sequence alone. Such information would allow the researchers to reduce the many steps involved in identifying mutations, recreating the mutations in strains, and observing how these mutations alter fitness when introduced into an organism. As a result of extensive laboratory evolution studies of E. coli B strain REL606 [1], Prof Barrick’s team has identified several commonly mutated genes within which a number of different mutations can confer fitness benefits.
We spoke to Dr Daniel Deatherage, a postdoctoral fellow in Prof Barrick’s lab, who is investigating the genetic diversity of these adaptive mutations. He and his colleagues work with 18 different bacterial populations, half of which are derived from REL606. The other half are from a similar strain, differing only in a mutation in the mutS gene, which makes them mutate at high frequency. The scientists included this hyper-mutating strain to ensure they would see sufficient mutations for their analyses. While insufficient mutations in REL606 did not turn out to be an issue, use of both strain backgrounds has enabled the group to compare the genetic diversity that arises as mutants compete in each type of population. Their preliminary observations were as expected—more mutations occurred early and more interesting competition events occurred in the hyper-mutating populations.
The genomic regions being studied by the scientists are not hotspots for mutations. Rather, on examining previously published sequencing data from 12 replicate E. coli REL606 populations evolved for 20,000 generations, Dr Deatherage identified genes that are consistently mutated among all replicates. Thus, in up to 12 independent experiments, a mutation in a specific gene was highly beneficial and able to sweep through the entire population. So the team was confident that under these conditions early mutations in these genes would arise in their next set of evolution experiments. By using target enrichment procedures they hoped to achieve adequate sensitivity to detect many competing mutations in these genes when the mutations were still “new” and very rare within the population.
Sequencing populations to identify rare mutations
To sequence the commonly mutated genes, researchers in the Barrick laboratory performed target enrichment of these gene regions using xGen Lockdown Probes from IDT. This allowed them to focus sequencing runs on only the regions of interest, enabling greater depth of coverage, reducing the amount of data collection and analysis, and saving on the cost of sequencing reagents.
The protocol started with growing successive generations of the 18 individual cell populations in liquid culture and transferring 100 μL culture into 10 mL fresh medium each day (approximately 6.7 generations per transfer). The cells were periodically plated during culture to monitor for contamination, and aliquots of the cells were frozen daily. A trick employed by the scientists was to inoculate each population with araA+ and araA– clones, which when grown on tetrazolium indicator agar, form white and red colonies, respectively. Use of araA+/araA– provided a phenotype that distinguished the 2 cell types in a population and enabled tracking of mutation frequency; e.g., when red colonies were no longer visible because they had been outcompeted by white colonies, the scientists knew that at least one mutation had swept through the population as a whole. The cells were passaged for ~500 generations (~75 passages).
Genomic DNA from the 500th generation of each population was isolated for enrichment and sequencing. An Illumina library preparation was made using custom adapters based on Illumina adapters, but with different barcoding sequences and other minor modifications. Approximately 120 xGen Lockdown Probes were then used to enrich 8 regions that contain the gene sequences of interest, following the Nimblegen SeqCap® protocol and using appropriate blocking oligos for the modified Illumina adapters. Hybridization was performed for 72 hours, followed by stringent washes with hard vortexing using reagents heated to 90°C. Dr Deatherage revealed, “The whole time I was purifying the DNA from the pulldown [target capture] I was thinking, “There is no way there is going to be anything left here.” I was vortexing so hard; but I had faith in what other people have done. Sure enough when we got the sequencing back, we had very nice coverage on almost all of our samples.” Subsequently, sequencing was performed on the enriched DNA using an Illumina HiSeq® instrument.
Sequencing outcomes
Dr Deatherage and colleagues identified populations of interest that they believed had higher frequencies of mutation at the endpoints (Figures 1 and 2). As a next step, the researchers will trace the progression of those populations using the frozen stocks to examine the frequency of mutations at closer intervals and to monitor the mutations as they rose to prominence, and possibly fell to extinction, in the populations over time. Ultimately, the scientists hope to correlate the increase in mutation frequency, when the mutations were new and ultra-rare, with their observed fitness effects. They are looking forward to eliminating competition assays, which are more tedious—it can take several weeks to create a mutant, and then 1 week to perform the assay.
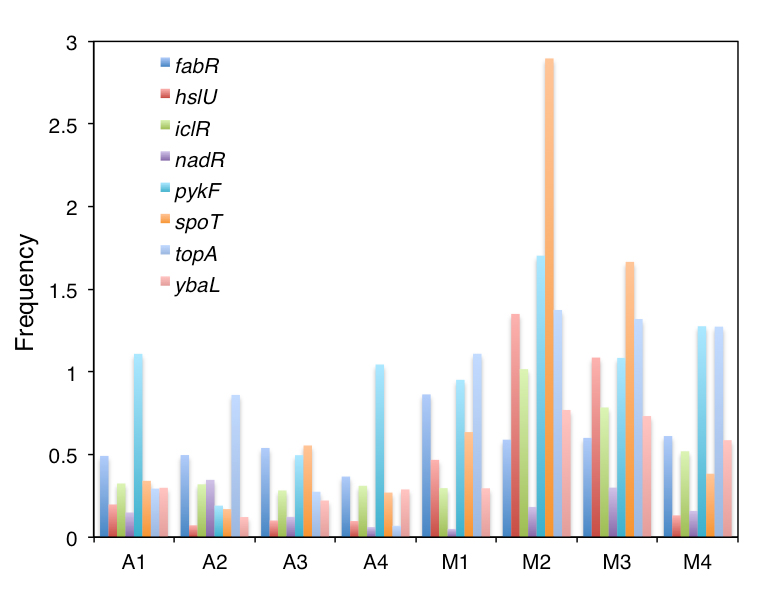
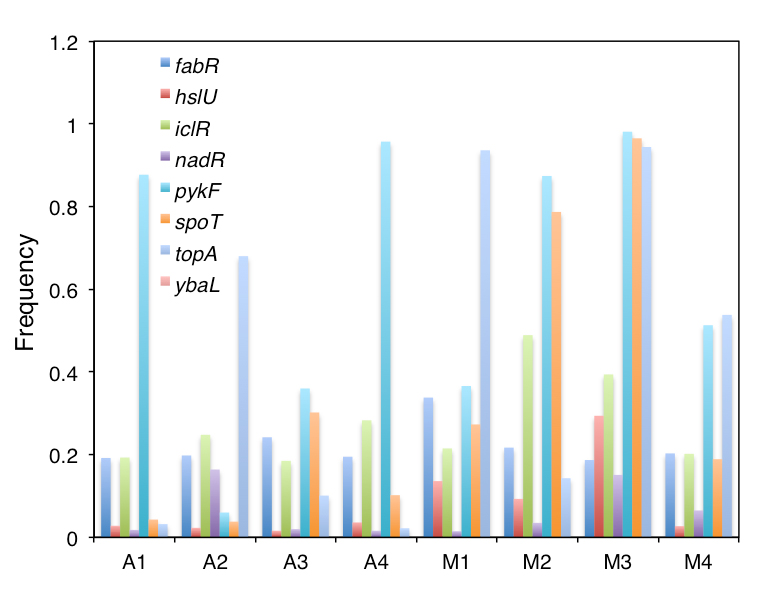
Dr Deatherage is excited about the idea of being able to get the same answers from sequencing. He stated, “Hopefully, one day we won’t even need competition assays; instead, we will be able to track the frequency of a mutation using targeted capture assays over a given time course and gauge its importance based on the fitness effect.”
Mutations confer fitness benefits
The scientists see evidence of clonal interference—multiple beneficial mutations within an asexual population competing with each other to take over the population. They also see evidence of multiple mutations within a single gene, and high frequency mutations in multiple genes within a single population, which raises the possibility that secondary mutations (rather than just a single mutation) are contributing to the fixation of mutations in a population. Dr Deatherage believes this process may be causing suboptimal mutations to fix in a population because if, for example, one mutation of topA provides a 10% fitness effect and another provides an 11% fitness effect, resulting in a difference of only 1% between the two, these mutations should coexist for many generations. While coexisting, both subpopulations will continue to evolve. If the subpopulation with the 10% fitness increase picks up a mutation in another gene, e.g., spoT, which confers an additional 10% fitness benefit, that cumulative fitness advantage of 20% will drive that subpopulation towards fixation markedly faster. The subpopulation with the 11% fitness effect from topA becomes in danger of extinction if it does not pick up another beneficial mutation, despite the topA allele conferring a superior fitness benefit on its own.
Data analysis challenges
Several challenges exist with analyzing the sequencing data. A major issue is the error rate current NGS sequencing methods incur, particularly when coupled with relatively low sequencing read coverage. Illumina’s reported error rate per base per read works out to 1 in 1000 bases. Thus, a base change present in 1 in 100 reads cannot be confidently classified as a mutation vs. an error in sequencing because this would be expected to happen by chance at many positions in the reference genome. However, an increased number of reads at this position (e.g., 100 identical errors in 10,000 reads), would allow one to confidently conclude that there was a mutation present at a frequency of ~1%. Deeper coverage can theoretically allow mutation calls to be made at frequencies approaching the error rate.
The team intends to further improve the confidence of their calls through the use of “duplex sequencing” [2]. Duplex sequencing works by using modified Illumina adapters that have 12 bases of random sequence at the 3’ end. These 12 random bases are sequenced as part of the read and can be used to identify all reads that arise from the same molecule of gDNA. By comparing all reads that correspond to a single molecule of DNA, consensus sequences can be produced confidently, eliminating errors that occur in a single read due to sequencing or PCR errors. Duplex sequencing reports a theoretical error rate of 1 per 1 billion bases of sequence.
xGen Lockdown Probes as the tool of choice for target capture
When Dr Deatherage started researching target capture for this project, he immediately eliminated chip-based methods where an excess of DNA is used to saturate the limited number of probes on the chip, resulting in recovery of only a portion of the DNA. He was concerned that this could lead to mutated sequences not binding as well as reference (wild type) sequences and, therefore, being selectively excluded from capture. Additionally, as most existing target enrichment panels were focused on human exome–sized enrichment, targeting at least 25 Mb (6X the size of the 4.6 Mb E. coli genome they were studying) was pointless. Dr Deatherage commented, “That is one very nice thing about the IDT probes—we could start from a single biotinylated probe and expand to a whole pool of these [xGen] Lockdown Probes. The targeted range was very nice.”
“Eventual winners, eventual losers” and cancer applications
Some members of Prof Barrick’s lab are interested in investigating so-called “eventual winner, eventual loser” situations, where one subpopulation can initially have a fitness advantage over a lesser subpopulation due to different mutations that each has accumulated, but after more time the lesser subpopulation has a better chance of experiencing more beneficial mutations and, therefore, reliably outcompeting the other population over the long term [3]. Dr Deatherage described this as a specific, highly beneficial mutation limiting the ability for further evolution in the losers. The researchers plan to examine additional cases of the eventual winner, eventual loser dynamic to understand the molecular mechanisms that lead to interactions between mutations that result in this “dead-end” effect.
Another application of this research is monitoring the evolution of cancer. Considering cancer to be an evolutionary disease with multiple mutations that allow the cells to escape growth restrictions, the team plans to monitor the evolution of tumors. The scientists can identify key oncogenes that are mutated in cancers, and then capture them with xGen Lockdown Probes for further study. They should then be able to monitor mutations in these targets at lower frequencies than is currently possible due to the large size of the human genome, a prospect Dr Deatherage finds very exciting.